Program
Program details
The preliminary program overview can be found here.
There will be three types of presentations: long oral, short oral, and poster.
Long oral presentations (full paper, around 45 slots):
- 25 minutes presentation by the author
- 15 minutes discussion by another student’s supervisor
- 10 minutes for questions and general discussion
Short oral presentations (around 20 slots):
- 15 minutes presentation by the author
- 5 minutes general discussion
Please find also some guidelines for presenters, session chairs, and discussants here.
Keynote
Keynote speaker
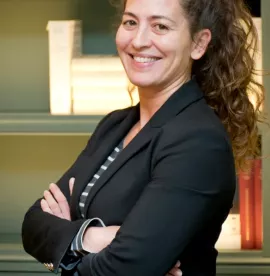
This year's keynote speaker will be Prof. Libertad Gonzalez, Professor of Economics at Universitat Pompeu Fabra and the Barcelona School of Economics.
"How family policies affect child health and development"
Workshops
Pre-conference workshops
There will be two pre-conference workshops, taking place on August 28.
Workshop 1 - Causal Machine Learning
Facilitator: Prof. Martin Huber, University of Fribourg
Description: This course provides an introduction to causal machine learning with applications using the software “R”. Causal machine learning aims at assessing the causal effect of some intervention or treatment, like offering or not offering medical treatment, on an outcome of interest, like health. The assessment of a causal effect requires that groups receiving and not receiving the treatment are comparable in background characteristics which also affect their health outcome (e.g., pre-treatment health, education, etc.). Causal machine learning can be used to generate such comparable groups in a data-driven way by estimating two separate models for how the characteristics affect the treatment and the outcome. Such approaches also permit detecting subgroups for whom the treatment effect is particularly large as a function of their observed characteristics (effect heterogeneity analysis). This is useful for optimally targeting specific subgroups by the treatment (optimal policy learning). The course discusses the underlying assumptions, intuition, and usefulness of machine learning for causal analysis. It also introduces various causal machine learning algorithms, like double machine learning and optimal policy trees. Using the statistical software “R” and its interface “R Studio”, these methods are applied to various real-world data sets.
Objectives: 1) To understand the ideas and goals of machine learning for causal analysis; 2) To understand the intuition, advantages, and disadvantages of alternative methods ; 3) To be able to apply causal machine learning to real-world data using “R” and “R Studio.”
Prerequisites: Introductory statistics (probability theory, conditional means, linear regression), basic command of the statistical software “R” is desirable, but not strictly required.
Workshop 2 - Academia or Beyond: Choose Your Health Econ Destiny
This interactive workshop will highlight different career paths for health economists.
Details will be announced soon.